Machine Learning to Predict AMD-Associated Genetic Variant Impact
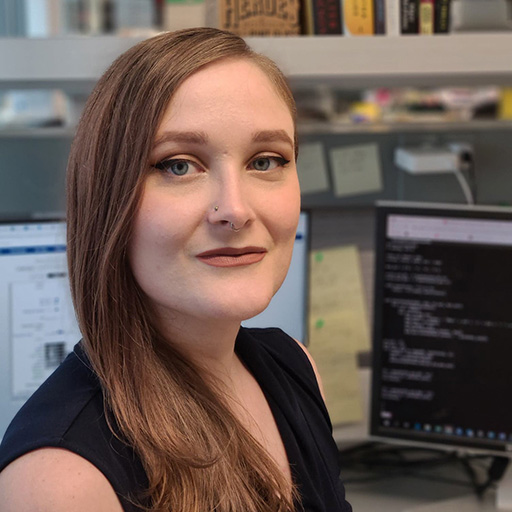
About the Research Project
Program
Award Type
Postdoctoral Fellowship
Award Amount
$200,000
Active Dates
July 01, 2022 - June 30, 2025
Grant ID
M2022006F
Goals
In this project, we aim to develop models that can accurately predict the potential impact of non-coding DNA sequence variants on the development of age-related macular degeneration (AMD).
Summary
In Aim 1, I will use genomic information to train machine learning models that are specific to retinal cell types, including the retinal pigmented epithelium. Following the generation of these models, in Aim 2 I will then use these models to predict the impact of sequence variations in relevant genomic regions, including variants that are known to be associated with AMD. Finally, in Aim 3 these predictions will be tested in cells to demonstrate the true impact in sequence activity with and without the predicted variants.
Unique and Innovative
This project marries innovative strategies including machine learning, organoid culturing, and next-generation sequencing to predict information about retinal genomic regulation. The models generated will be useful in prioritizing non-coding variants that may contribute to AMD and have thus far been understudied. As a result, AMD-associated variants can be prioritized for functional follow up and variant prediction scores can be benchmarked for future use in genetic testing.
Foreseeable Benefits
The final validated models developed from this study will be able to accurately predict the impact of sequence variations specifically in relation to AMD. Because of this, novel sequence variations identified in AMD patients will be able to be quickly and easily queried to identify those variants which could be pathogenic. This could allow for new treatments to become more quickly available based on known pathogenic variants.
Grants
Related Grants
Macular Degeneration Research
The Role of Mitochondrial Dysfunction in Age-Related Macular Degeneration
Active Dates
July 01, 2023 - June 30, 2025
Principal Investigator
Navdeep Gogna, PhD
The Role of Mitochondrial Dysfunction in Age-Related Macular Degeneration
Active Dates
July 01, 2023 - June 30, 2025
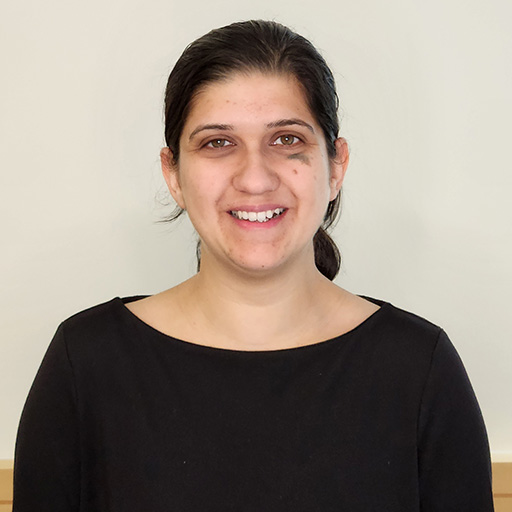
Principal Investigator
Navdeep Gogna, PhD
Macular Degeneration Research
Mouse Models for Subretinal Fibrosis
Active Dates
July 01, 2022 - June 30, 2025
Principal Investigator
Patsy Nishina, PhD
Mouse Models for Subretinal Fibrosis
Active Dates
July 01, 2022 - June 30, 2025
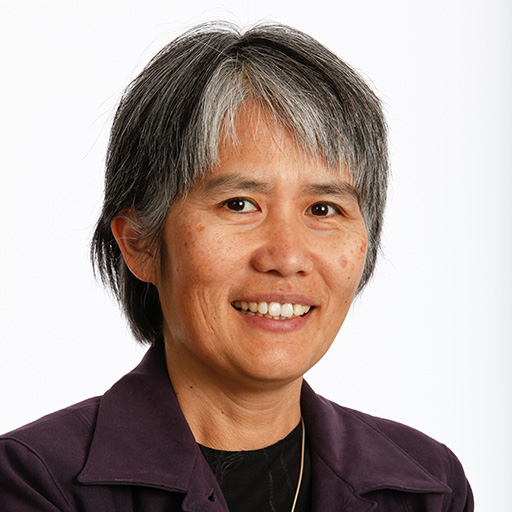
Principal Investigator
Patsy Nishina, PhD
Macular Degeneration Research
Gene Regulation of RPE Maintenance
Active Dates
July 01, 2022 - June 30, 2025
Principal Investigator
Lev Prasov, MD, PhD
Gene Regulation of RPE Maintenance
Active Dates
July 01, 2022 - June 30, 2025
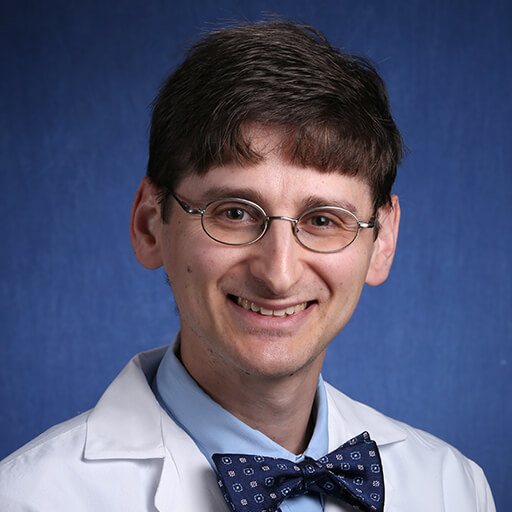
Principal Investigator
Lev Prasov, MD, PhD