A Novel Approach to Personalized Prediction of Progression of Age-Related Macular Degeneration

About the Research Project
Program
Award Type
Standard
Award Amount
$198,052
Active Dates
July 01, 2019 - December 31, 2023
Grant ID
M2019155
Co-Principal Investigator(s)
Daniel Rubin, MD, MS, University of Illinois Urbana-Champaign
Luis Sisternes, PhD, University of Illinois Urbana-Champaign
Theodore Leng, MD, FACS, University of Illinois Urbana-Champaign
Goals
The majority of patients with advanced AMD have severe vision loss. Despite the development of artificial intelligence algorithms for personalized AMD progression, we are still far from implementing tailored follow-up care and treatments for AMD patients. Our hypothesis is that the probability of AMD progression can be predicted by integrating imaging, genetic and clinical data in statistical predictive models, thereby improving personalized care.
Grantee institution at the time of this grant: University of Illinois Urbana-Champaign
Summary
The goal of our study is to predict the progression of age-related macular degeneration (AMD). We will first expand and validate our fully-automated image processing algorithms in predicting future choroidal neovascularization and geographic atrophy events to include genetic data and real-world longitudinal patient data. In our second aim, we will initiate a randomized, controlled clinical trial to test the viability of applying personalized AMD prediction models. This study integrates imaging, genetic, demographic, and clinical patient parameters from several data sources, with an existing pipeline for image feature extraction. This innovative approach will provide time-dependent probabilities for predicting advanced AMD. Testing algorithms in a clinical trial setting is another novel aspect of this research.
Our work will advance the AMD field by improving the identification of high-risk patients as candidates for more frequent screening and earlier treatment, leading to better clinical outcomes. Results may lead to developing a tool to predict the chances of AMD progression on a personalized, patient-by-patient basis.
Grants
Related Grants
Macular Degeneration Research
Innovative Night Vision Tests for Age-Related Macular Degeneration
Active Dates
July 01, 2024 - June 30, 2027
Principal Investigator
Maximilian Pfau, MD
Innovative Night Vision Tests for Age-Related Macular Degeneration
Active Dates
July 01, 2024 - June 30, 2027
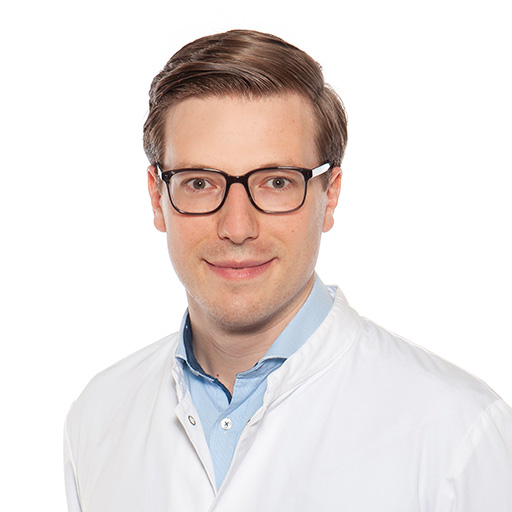
Principal Investigator
Maximilian Pfau, MD
Macular Degeneration Research
The Development of a Transplant-Independent Therapy for RPE Dysfunction
Active Dates
July 01, 2024 - June 30, 2026
Principal Investigator
Shintaro Shirahama, MD, PhD
The Development of a Transplant-Independent Therapy for RPE Dysfunction
Active Dates
July 01, 2024 - June 30, 2026
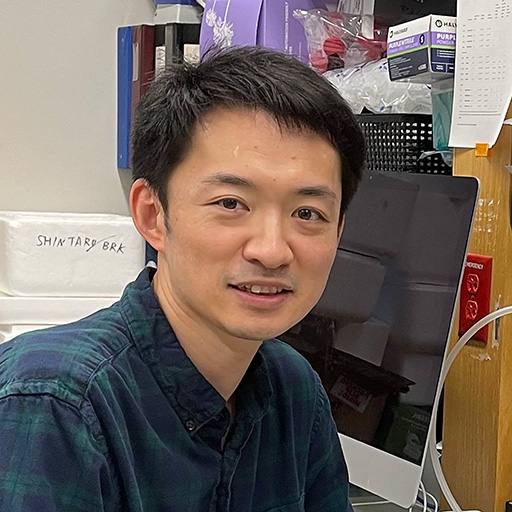
Principal Investigator
Shintaro Shirahama, MD, PhD
Macular Degeneration Research
Immune Cell Traps in Inflammation and Wet Age-Related Macular Degeneration
Active Dates
July 01, 2023 - June 30, 2026
Principal Investigator
Matthew Rutar, PhD
Immune Cell Traps in Inflammation and Wet Age-Related Macular Degeneration
Active Dates
July 01, 2023 - June 30, 2026
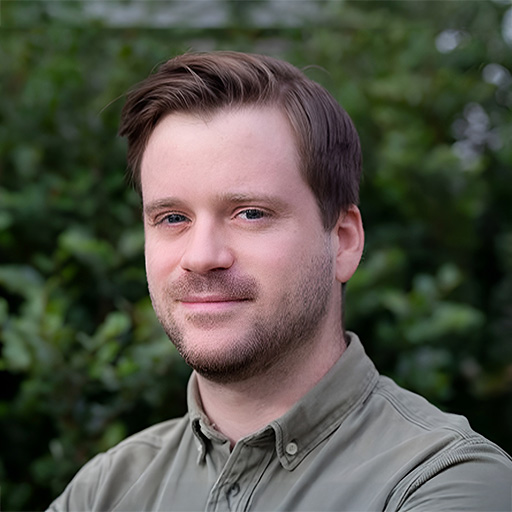
Principal Investigator
Matthew Rutar, PhD